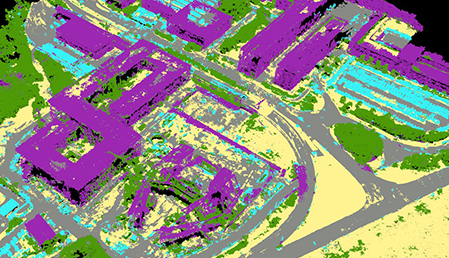
Pix4D has introduced a new image classification technique as part of its Pix4Dmapper photogrammetry software. The solution uses new machine vision techniques for photogrammetry for automatically classification of drone-based point clouds.
While Pix4D software is mostly known for photogrammetry from drone-captured imagery, one of its latest mapping innovations is a software feature that automatically classifies drone-based point clouds, based on machine learning techniques. By using this feature, entire 3D point clouds are classified into individual groupings, divided into categories such as buildings, roads or vegetation.
The solution has recently been integrated in Pix4Dmapper, the company’s photogrammetry software product for drone-based mapping and automatically classifies 3D points based on both geometry and color. Ideally, a situation would exist where raw imagery would be converted automatically by the software into 3D models with attributed semantic information, but this new feature is a significant step in that direction and introduces machine learning tools in photogrammetric workflows.
At this moment, the feature distinguishes between five different categories: buildings, high vegetation, human-made objects, roads and ground. Before, this classification took a lot of time and had to done manually by isolating the data of interest: now, the company claims 10 million points can now be classified in 3 minutes time. These will be used for automatically generate DTM´s, meaning a surface model without any (natural or man-made) objects. This explains why the classification scheme includes high vegetation (for example trees), which are removed when a DTM is created.
In the near future, this classification scheme will be developed and refined further, so that buildings can be extracted and modeled as a semantic composition of geometry, including elements such as roofs, facades, windows, doors and balconies. Other applications that benefit from the classification scheme are volume measurement and vegetation growth control.
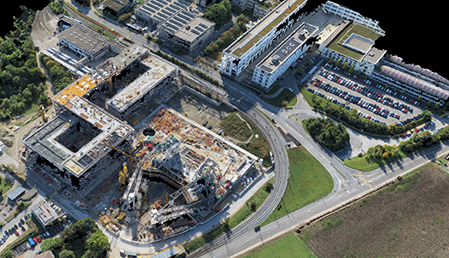
Machine-learning technology refers to software algorithms that refine themselves by processing more data, a famous example being Google´s search algorithms that get refined over time, taking the searches of users as input to this process. Pix4D employed the same technique by giving its algorithms test data and telling what the data represented visually, in order to apply the classification system described above and over time, the system got better and quicker after a “learning process” of more training data. Pix4D users were also given the option to help with this process. Finally, the classification feature was added to the Pix4Dmapper software.
Each individual point in a 3D point cloud that is generated through Pix4D’s software is referenced to all the images used to calculate it, providing extra information to the machine learning process and can be used for more realistic 3D visualizations, analysis and measurement that can be used for applications that previously relied on stereo-pair matching. This technique is called rayCloud and is also integrated into Pix4Dmapper.
One area that can benefit from the new classification feature is the construction surveying industry, realizing time and cost savings over terrestrial laser scanning. Apart from point cloud classification, the Pix4Dmapper software also offers functionality to manually edit point group classifications, adding or removing selected points, adding new groups and more. The Pix4D point cloud data can also be exported to Autodesk or Bentley software, as a LAS file including the classifications or no more than a subset of the classifications to software that doesn´t recognize the LAS data format.
Comments